AI, Explain Thyself
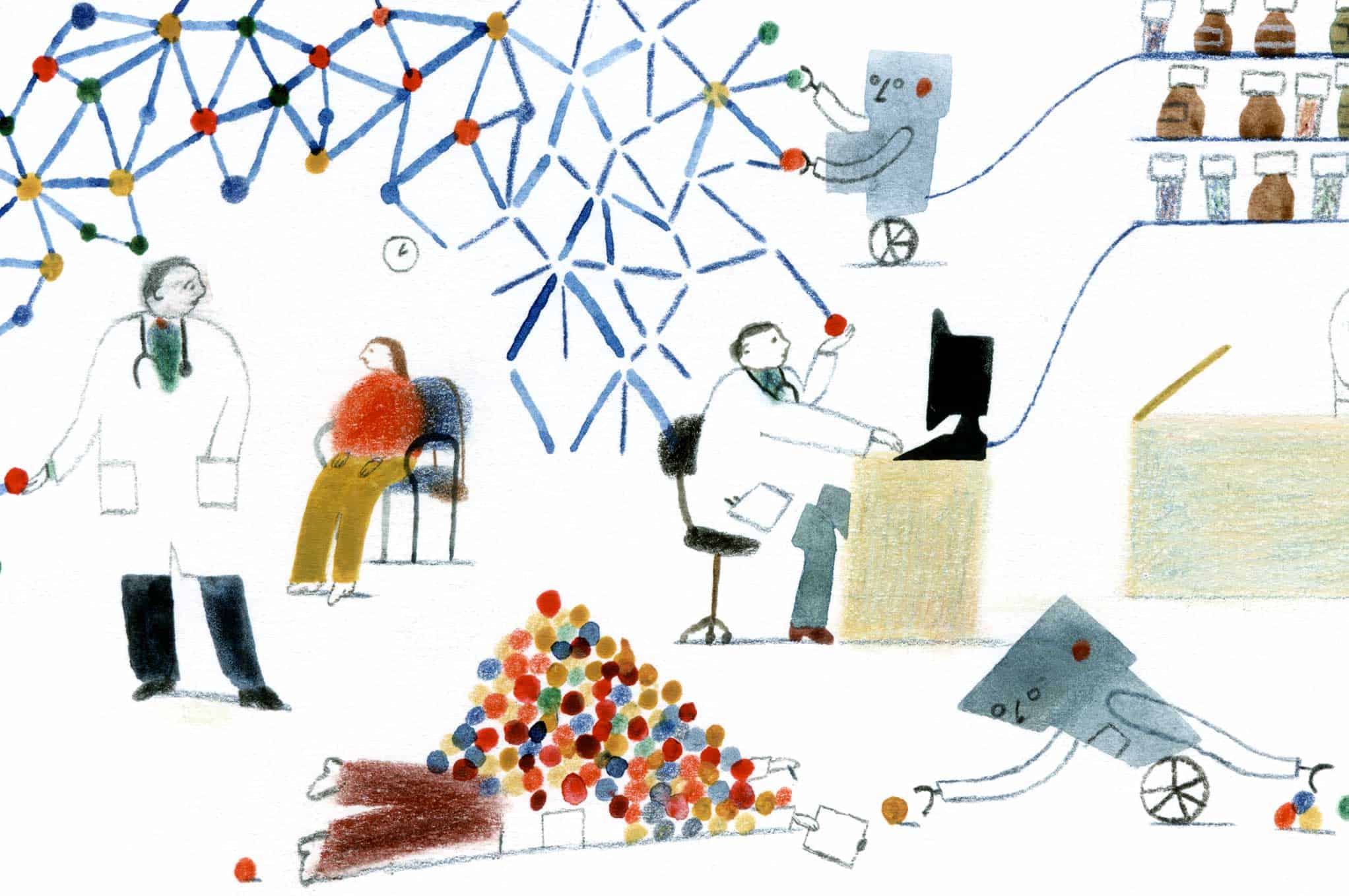
PUBLISHED NOVEMBER 20, 2021
The past decade has seen machine learning—finding patterns in vast piles of data—in a state of vibrant growth. The number of life science papers on machine learning numbered just under 600 in 2010; by 2019, there were more than 12,000. The applications in medicine are potentially lifesaving and include the ability to help physicians home in more quickly on the right diagnosis (“Doctors in the Machine,” Winter 2015).
The FDA has already approved 29 medical devices that use machine learning in some way, with dozens of others in the pipeline. Translational research teams are also looking to find room in clinical practice for an astonishing range of its insights, including which patients are most likely to miss an insulin dose and who might attempt suicide in the next six months.
But there is a downside. Human researchers are, by and large, unable to follow the logic behind many of these algorithms—including almost all of those used in FDA-approved technologies. The insights are created by passing the information through “hidden layers” of complex networks to develop predictive patterns—a black box approach where the logic becomes opaque.
“To say that an algorithm is a black box means that it wouldn’t be interpretable even by the people who designed it,” says Boris Babic, a professor of philosophy and statistics at the University of Toronto. The parameters and their relationships become so complicated, he says, that it becomes mathematically impossible to piece together how the inputs lead to the outputs.
Some might argue: So what? If the algorithms have predictive power, then let the black box be black. But others are concerned about dangerous assumptions that machines might cook up or “catch” from the data they import. Where a tool is learning from human example, for instance, it might perpetuate existing biases—clinicians’ tendencies to take women’s accounts of pain less seriously than men’s, for instance.
Researchers and policymakers have increasingly called for algorithms that can explain what they’re doing. The U.S. National Institute of Standards and Technology held a workshop earlier this year to lay out new benchmarks. The Royal Society issued a policy brief in favor of explanations, and the European Union, after it passed the General Data Protection Regulation in 2016, has increasingly advocated a “right to explanation” about the algorithms that affect people’s lives.